Data Granularity
Data granularity refers to the level of detail or specificity at which data are collected, stored, and presented. It can apply to various types of data, such as time, geography, or other attributes. Fine-grained data, like Census tracts or block groups, may provide more detail, whereas coarse-grained data is aggregated at a higher geographic level, like county or regional level data. More detailed, granular geographic data may provide more accurate, nuanced analysis and interpretation. It enables data users to identify trends, patterns, and anomalies that might be missed when working with coarse-grained data.
When selecting the level of granularity, trade-offs must often be made. For example, sometimes one must choose between a coarse-grained geographic dataset (e.g., data at the county-level) that includes population breakouts like race and ethnicity, or a finer grained geographic dataset (e.g., data at the census tract level) that does not provide race or ethnicity breakouts because it has too small of a sample to provide valuable demographic insights.
When exploring indicators for your community, you will encounter data available at varying levels of detail—some data offers finer granularity, while others are coarser-grained. In some cases, you may not have a choice for how to explore the data, as it may only be available at one level (e.g. data for each state). When accessible, determining at what level to explore data often depends on the area of interest and the level at which data-driven actions or decisions will be made. For instance, when making decisions at the federal level, using neighborhood-level information could be overly detailed; on the other hand, county- or state-level data might be insufficient for hyperlocal decision-making. Choosing the appropriate granularity level ensures that decisions are aligned with your goals.
One common example of the challenge of data granularity is in rural areas. Coarse-level county data encompasses both rural and urban areas, and specific insights about the rural areas are difficult to suss out. In these cases, a finer-grained dataset that distinguishes between urban and rural areas could be more informative.
Relying solely on coarse data for decision-making can lead to misinterpretation or inaccurate assumptions, like masking disparities. However, using the finer-grained localized data, you might notice that there are pockets of very low rates of affordable housing which were masked or smoothed out when looking at the county as a whole. While not always available, fine-grained data can mitigate misunderstandings and provide more clarity. Having fine-grained data allows for a more accurate representation of diverse populations, which is especially important for exploring data across populations that are historically marginalized. Fine-grained data can reveal disparities and inequities that might not be apparent in aggregated data. When assessing issues related to race, gender, socioeconomic status, or other equity-related factors, fine-grained data can help identify areas of concern and enable targeted interventions. In some cases, fine-grained data might contain sensitive information that could potentially harm individuals or communities if mishandled. Striking a balance between using granular data and protecting privacy is essential.
Resources & Tools
A Scoping Review of Virtual Focus Group Methods Used in Rehabilitation Sciences
Resource - Journal Article
Using the Methods of the Public Health Disparities Geocoding Project to Monitor COVID-19 Inequities and Guide Action for Health Justice
Resource - Website/webpage
Brought to you by Harvard University T. H. Chan School of Public Health
More Than Numbers: A Guide Toward Diversity, Equity, and Inclusion (DEI) in Data Collection
Resource - Guide/handbook
A Guide to Conducting Online Focus Groups
Resource - Guide/handbook
Brought to you by Vital Strategies
Focus Group Tip Sheet
Resource - Fact Sheet
Brought to you by U.S. Department of Health and Human Services
Perils and Possibilities: Achieving Best Evidence from Focus Groups in Public Health Research
Resource - Journal Article
Understanding and Interpreting Population Health Measures
Story - Written
Brought to you by IP3
Published on 11/15/2022
Related Topics
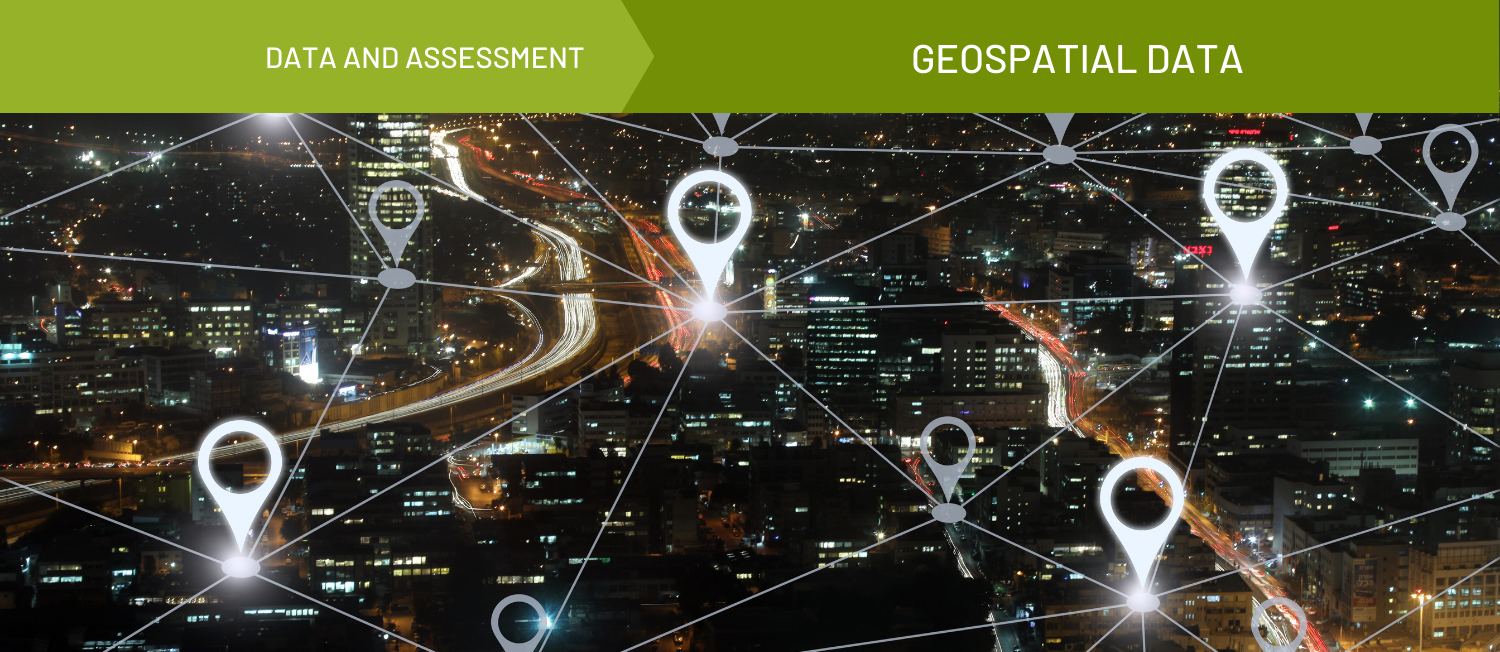
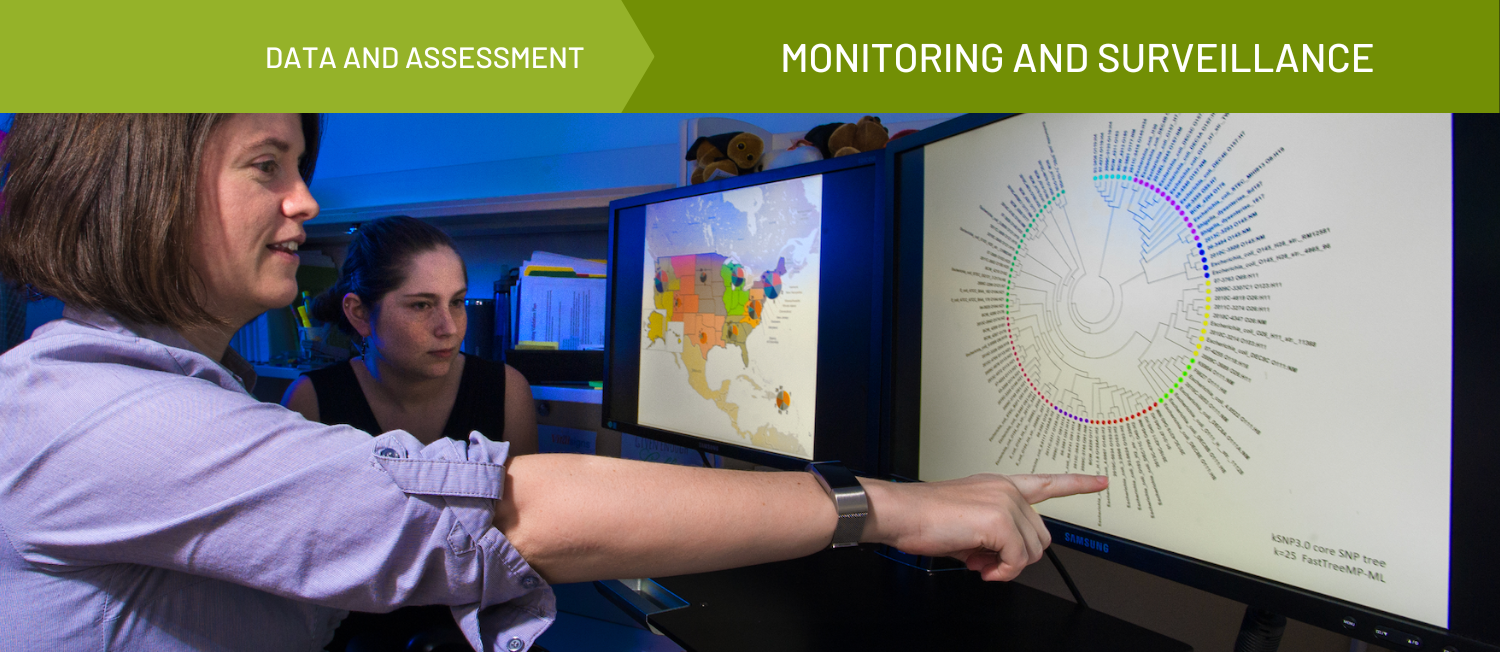
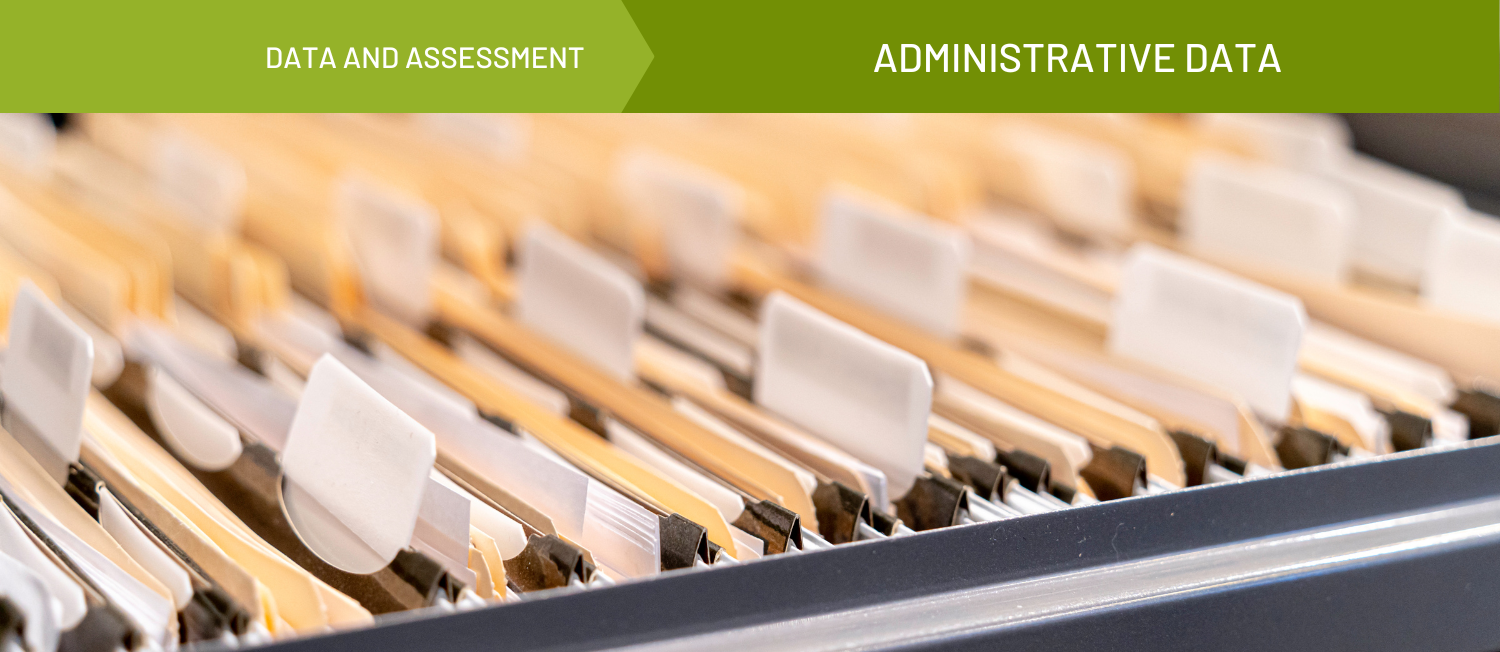
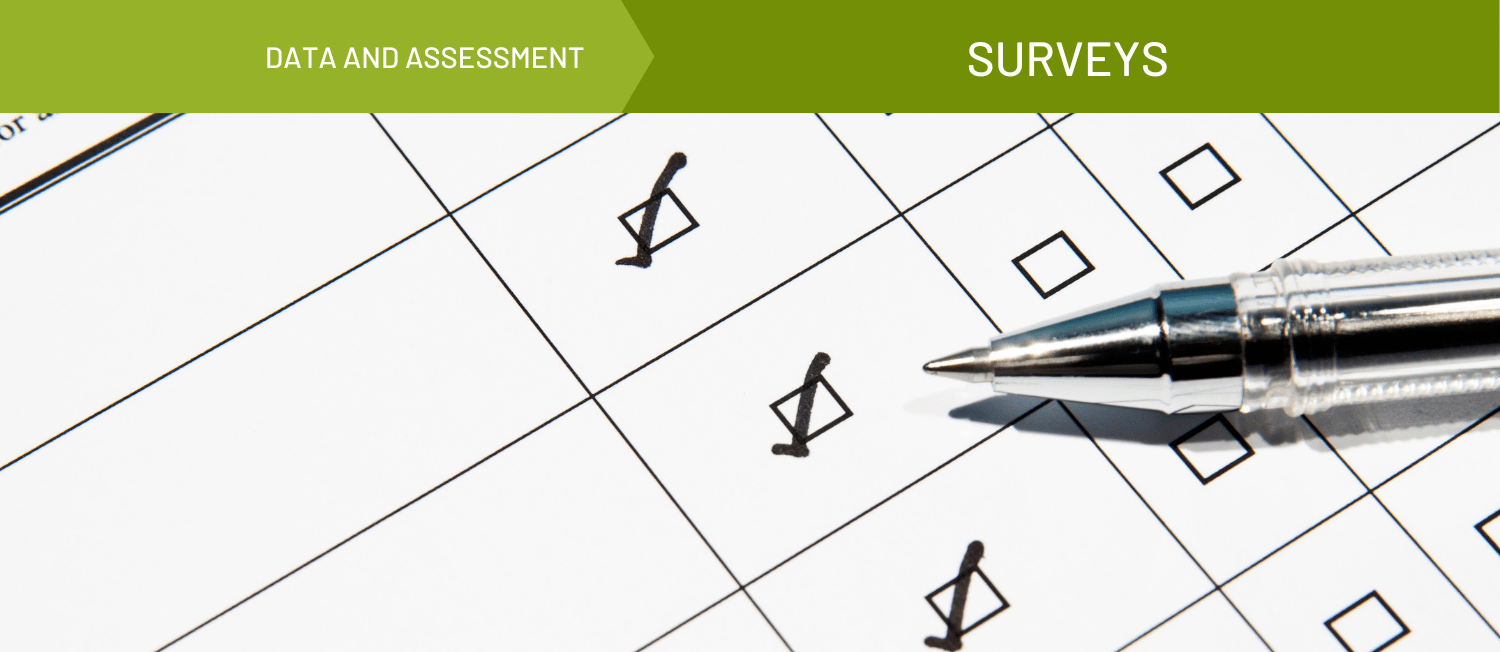
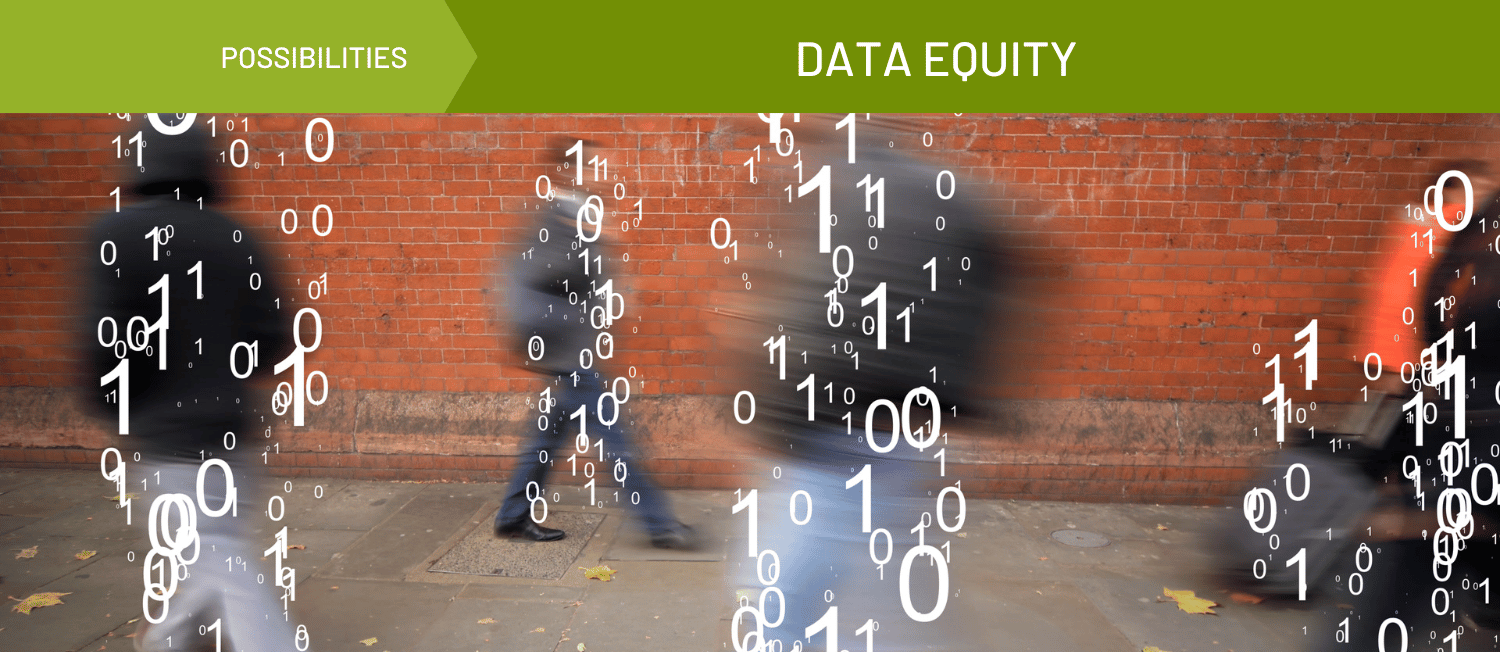
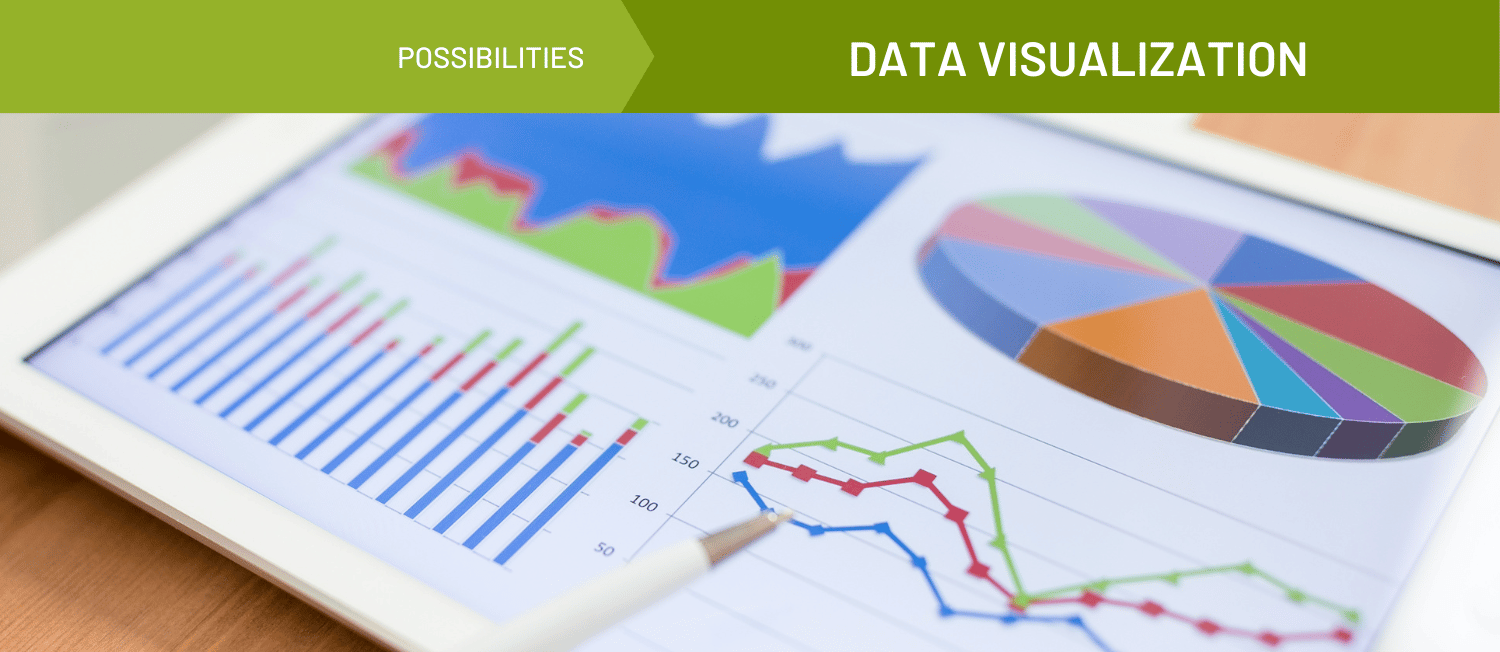
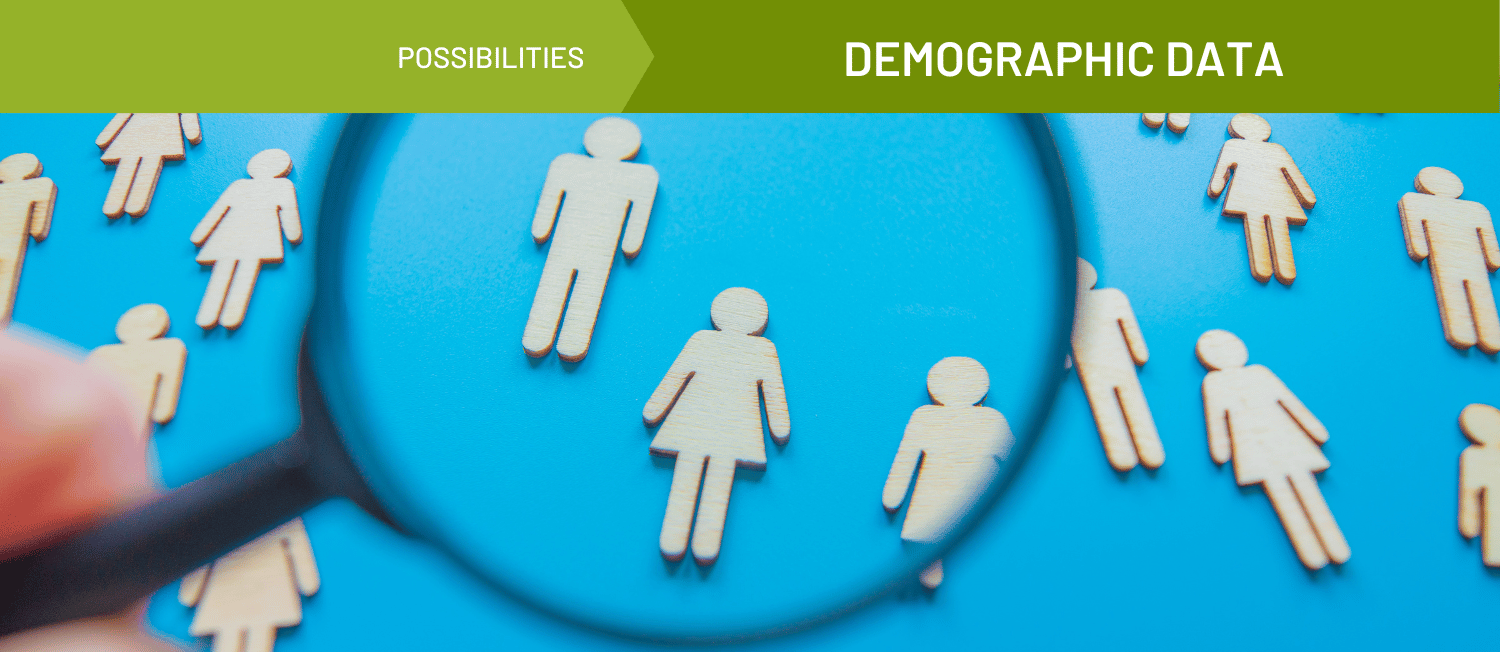